An Interview with Tenstorrent: CEO Ljubisa Bajic and CTO Jim Keller
by Dr. Ian Cutress on May 27, 2021 11:45 AM EST- Posted in
- CPUs
- AI
- Jim Keller
- ML
- 100G Ethernet
- Tenstorrent
- Wormhole
- Ljubisa Bajic
- Grayskull
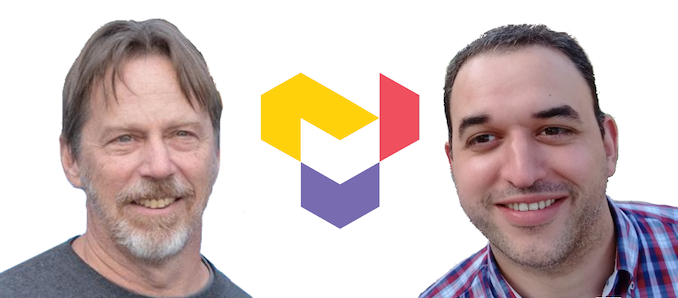
Many billions have been poured into the industry when it comes to AI processor development. If you were to list the number of AI processors currently in development or production at the wide variety of start-ups, then that number quickly surpasses 50 and is seemingly forever rising. Each one of these companies is aiming to build a compelling product to meet the needs of their intended customers, all the way from small-scale inference workloads up to multi-datacenter scale training. One of these companies is Tenstorrent, led by CEO Ljubisa Bajic, who recently hired famed chip designer Jim Keller as the CTO. Jim was also the initial angel investor when the company started. Today we are interviewing these two about the company, the Tenstorrent product, and the direction of the demands for machine learning accelerators.
This interview was somewhat unexpected – about a month ago Jim Keller reached out to me to ask if we wanted to interview him and Ljubisa about the company. We had highlighted at the start of the year when Jim was hired by Tenstorrent, and I’ve sat through a number of Tenstorrent presentations by Ljubisa and his team, so whatever company Jim lands at gets a lot of attention. Apparently my name was recommended to reach out for an interview, and here we are!
We actually split the interview into two segments. This is the first 90-minute interview transcription, on the topic of Tenstorrent with Ljubisa and Jim. There will be a second 90-minute interview to follow, on the topic of Jim Keller, next week.
![]() Ljubisa Bajic CEO Tenstorrent |
![]() Jim Keller CTO Tenstorrent |
![]() Ian Cutress AnandTech |
Ljubisa Bajic is a veteran of the semiconductor industry, working extensively in VLSI design and debug coupled with extensive accelerator architecture and software experience. Ljubisa spent a decade inside AMD as an ASIC architect working on power management and DSP design, before a spell at NVIDIA as a senior architect, and then back to AMD for a couple of years working with Jim on the hardware that powers AMD’s balance sheet today. He left AMD to start Tenstorrent with two other co-founders in 2016, has raised $240m in three rounds of funding, and created three generations of processors, the latest of which is shipping this year. At least two more generations are on the way.
Jim Keller has often been described as a hardware ninja, or a semiconductor rockstar. His career is full of successful silicon designs, such as the DEC Alpha processors, AMD K7/K8, HyperTransport, Apple’s A4/A5 processors, AMD Zen family, Tesla’s Fully Self-Driving chip, and something important at Intel (probably). He now sits as CTO for Tenstorrent, working on the company’s next-generation processors for 2023 and beyond.
Tenstorrent is a pure-play fab-less AI chip design and software company, which means that they create and design silicon for machine learning, then use a foundry to make the hardware, then work with partners to create solutions (as in, chips + system + software + optimizations for that customer). For those that know this space, this makes the company sound like any of the other 50 companies out in the market that seem to be doing the same thing. The typical split with pure-play fabless AI chip design companies is whether they are focused on training or inference: Tenstorrent does both, and is already in the process of finalizing its third-generation processor.
IC: Who or what is Tenstorrent, in your own words?
LB: Tenstorrent is a start-up company working on new computer architectures that are aiming to essentially be the right substrate for executing machine learning workloads. More data-heavy workloads have been emerging and taking over, especially the datacenter computing scene, but we want to go wider than that as well.
IC: Jim, you were the first investor in Tenstorrent - I remember you telling me a little while ago, when you joined the company five months ago. What is it about Tenstorrent that’s really got you excited and interested?
JK: So I know Ljubisa, and I’d seen a lot of AI start-ups. When I was at Tesla, everybody came to talk to us and pitch their stuff, and there seemed to be an odd collection of odd ideas. [There were] people who didn’t really get the full end of chip design, the software design, and all of that. I know Ljubisa – he has actually worked on GPUs in real chips and he ran a software team, and he actually understands the math behind AI, which is a somewhat rare combination. I actually told him, I thought it would be funny if he had a couple of guys working in a basement for a year, and so I gave him what’s called the Angel Round of investment, and they actually did. You were in a basement or were you over a garage or something?
LB: We were in a basement!
JK: You were literally in a basement so, and they built a prototype using an FPGA that got the seen round, and then that got him further to get the first day round.
IC: So was the basement in Toronto, as far as I know, you’re based in Toronto?
LB: Yes, it was the basement of my house, essentially the same basement I’m sitting in right now. I’ve come full circle, five years later!
JK: And the other thing I told him - make sure whatever you’re building always has software to run on it. There have been a number of AI efforts where they build a chip, and then they say ‘now we’re going to build the software’. They then find out the hardware/software interface is terrible, and it’s difficult to make that work. I think [Tenstorrent] did an interesting job on that.
I was talking to our compiler guys recently and they’re doing the third re-write of the software stack, which is actually great because the hardware is pretty good for software. They’ve had ideas, and it got to a certain point, and then they re-wrote it. The new rewrite is actually beautiful, which I think in the world of AI software, there’s not much beautiful AI software down that talks to the hardware.
IC: To come back to Ljubisa and the basement - Tenstorrent has three co-founders, was it just the three of you in those early days?
LB: So it was two of us for the first few months, then our third co-founder joined us right around that time Jim made the investment into Tenstorrent, kind of making the whole thing a little more legitimate, and a little bit less of a pipe dream. So a few months after that, we moved out of the basement and got an office which was again, a very typical, a very low-end office in a bad part of Toronto with shootings.
JK: Shootings in Toronto?
LB: Yeah, look for a bad part of town in Toronto! We were there for a while, it was a lot of fun in retrospect.
IC: So you’d spent the best part of a decade at AMD, a little time at NVIDIA, what made you decide to jump and form your own start-up?
LB: Well I really wanted to build a next-generation computer which was going to leave a dent of some kind on the computer scene. The problem materialized, which was machine learning. So I started Tenstorrent in 2016, and by then it was clear that this was a huge workload, that it was going to be a major upheaval, that it was going to be an internet grade revolution to our common space.
It’s difficult to make large departures off of what’s already there at big companies. That’s part of it. The other part of it was that due to completely unrelated reasons, I decided to move on and see what to do. You put the two together and at some point Tenstorrent got conceived of as an idea. It was very wobbly at first, so the first month or two I was thinking about it. I was very uncertain that it was really going to go anywhere, and the thing that really kicked it over was that Jim showed trust in the idea. He showed trust in my ability to do such a thing, like really in a very real way. If Jim had not invested, I don’t think we would have really gone forward. Probably I would have just gone on to get another job.
IC: So the two of you met together at AMD, working on projects together. I’ll start with you Jim, what was your first impression of Ljubisa working with him?
JK: Ljubisa is a big hulking guy, so we’re in this meeting and you know, all these nerdy engineers are talking and there’s Ljubisa saying this is how we should do stuff. He has, let’s say, a fairly forceful personality and he’d done a whole bunch of work on improving power efficiency of GPUs. When he first proposed that work, there was a bit of pushback, and then he slowly worked it out and he was right. I think Raja Koduri actually told him later on that a lot of the power/performance improvement they got came from the work he did.
Then he took over the team of software power management and system management, which had been sort of put together from a couple of different groups and it wasn’t very functional. Then he did a fairly significant transformation of that in terms of the charter, and also effectiveness. So I was kind of watching this go on.
When I was at AMD, the products they had (at the time) weren’t very good, and we literally canceled everything they were doing and restructured stuff and created a bunch of clean slate opportunities. Zen was, at the top level, literally a clean slate design. We reset the CAD flows, Ljubisa was resetting the power management strategy and a couple of other things, so he was one of my partners in crime in terms of changing how we do stuff. I don’t think you were a senior fellow, I found the best senior fellows at AMD, at least I thought they were the best, and they worked for me. I had a little gang, and then Ljubisa joined that gang, because everybody said he’s one of us. That was pretty cool, and then together we could basically get any technical problem moving because we had pretty good alignment, so that was really fun.
IC: Same question to you Ljubisa. Jim is a well-known name in the industry for semiconductor design, and he came into AMD kind of laying down the hammer – to rip everything up and start a clean slate. What was your impression of him at that point?
LB: On my second chart at AMD, I re-joined the company having explicitly decided that I was going to essentially apply whatever energy I’ve got into fixing everything in my sight, and balk at nothing. I joined with that mindset, and I didn’t know Jim at the time. But pretty quickly we intersected and also it became pretty clear to me that on my own, regardless of my enthusiasm and design to make a lot of impact, it was going to be difficult to get around all the obstacles that you generally come upon when you want to affect a lot of change in an organization of that size.
My first impression was that Jim was essentially absolutely bulldozing (Ian: Ha!) through anything that you could characterize as any kind of obstacle, whether it was like organizational or technical, like literally every problem that would land in front of him, he would just sort of drive right over it with what seemed like no sort of slowdown whatsoever. So given my disposition of what he was already doing, I think that’s ultimately, at least a part of, what led us getting into alignment so quickly and me getting into this group that Jim just mentioned.
I wasn’t a Senior Fellow, I was actually a director - everybody at the time kept saying that nobody understands why I’m only a director and why am I not a fellow, or a senior fellow. That was a common theme there, but I guess I fit in more with these technical folks and they said there are a lot of organizational challenges to getting anything serious done. I thought that it was better [for me to be] positioned somewhere you have a bit of reach into both.
For me the biggest initial impression was that Jim enabled everything that I wanted to do, and basically recognized and he did this for anybody that was in his orbit. He’s extremely good at picking people that can get stuff done versus people that can’t, and then essentially giving them whatever backing they need to do that.
As we started together, he started giving me all sorts of random advice. A story that I’ve mentioned before is that we had a meeting in Austin one time, and I was supposed to fly on Tuesday morning. I went to check-in early and realized that I had booked the ticket for a week earlier. So I never went to the airport, I never had a hotel, I didn’t have a flight. I called up Jim and I said ‘I got to buy another ticket and I can’t go through the corporate systems because I need to buy it now and the flight is 6am the next morning’. So he goes ‘yeah, you should really watch out for that - you’re kind of too young for this sort of behavior!’. I’ve gotten all sorts of life advice from him which I’ve felt was extremely useful and impactful for me. I’ve changed major things in the way I go about doing stuff that’s got nothing to do with computers and processors based off of Jim’s input. He’s been a huge influence – it started with work, but it goes deeper than that.
IC: Correct me if I’m wrong, but for the time inside AMD, it kind of sounded like Jim’s way or the highway?
JK: I wouldn't say that! The funny thing was, we knew we were kind of at the end of the road - our customers weren’t buying our products, and the stuff on the roadmap wasn’t any good. I didn’t have to convince people very much about that. There were a few people who said ‘you don’t understand Jim, we have an opportunity to make 5%’. But we were off by 2X, and we couldn’t catch up [going down that route]. So I made this chart that summarised that our plan was to ‘fall a little further behind Intel every year until we died’.
With Zen, we were going to catch up in one generation. There were three groups of people - a small group believed it (that Zen would catch Intel in one generation); a medium-sized group of people that thought if it happens, it would be cool; then another group that definitely believed it was impossible. A lot of those people laughed, and some of them kind of soldiered on, despite this belief. There was a lot of cognitive dissonance, but I found all kinds of people that were really enthusiastic.
Mike Clark was the architect of Zen, and I made this list of things we wanted to do [for Zen]. I said to Mike that if we did this it would be great, so why don’t we do it? He said that AMD could do it. My response was to ask why aren't we doing it - he said that everybody else says it would be impossible. I took care of that part. It wasn’t just me, there were lots of people involved in Zen, but it was also about getting people out of the way that were blocking it. It was fun – as I’ve said before, computer design needs to be fun. I try to get people jazzed up about what we’re doing. I did all kinds of crazy stuff to get people out of that kind of desultory hopelessness that they were falling into.
IC: Speaking of fun, in your scaled ML talk, there were a lot of comments on there because you used Comic Sans as the font.
JK: A friend of mine thought that it would be really funny, and to be honest, I didn’t really know why. But I’ve gotten a lot of comments about that. One of the comments was that ‘Jim’s so bad-ass that he can use Comic Sans’. I just thought it was funny. It was good because they didn’t tell me who I was presenting to - I walked in the room and it was all bankers and investors and university bureaucrats. They told me it was a tech talk and I thought ‘Oh, here we go!’.
IC: The title of your talk was ‘TBD’, I thought that was sort of kind of like an in-joke – ‘it’s the future of compute, so let’s call the talk TBD’?
JK: I think that might have been an in-joke!
IC: But speaking of something fun, so for Tenstorrent in 2016. At the time Jim was working on Tesla, on the self-driving stuff - when you were speaking to Ljubisa? At the time did Ljubisa have a concrete vision at that point? Was there something more than just who Ljubisa is, you know, gave you the impetus to invest?
JK: There were a couple of things. One was that we were all in that process of discovery about just how low the precision of the mathematics you needed to make neural networks work. Ljubisa had come up with a fairly novel variable-precision floating-point compute unit that was really dense per millimeter. He also had an architecture of compute and data, and then how he wanted to interconnect it which I thought was pretty cool. He had pretty strong ideas about how that worked. Pete Bannon and I talked to him a couple of times while we were at Tesla. The engine Ljubisa was building was actually more sophisticated than we (at Tesla) needed.
Pete was the architect of the AI engine in the Tesla chip, and it’s brilliant for running Caffe - just amazing. Half of the compiler team for that chip was Pete! It’s because, again, the hardware-software match was so good, and Caffe produced a pseudo instruction set which you trivially modified to go run on the AI engine. What Ljubisa was doing was more sophisticated, to run lots of different kinds of software.
Ljubisa at the time was already aiming at PyTorch I think? That was before PyTorch was the winner, at the time when TensorFlow was leading back then.
LB: At that time PyTorch was just called Torch still, and TensorFlow had just kind of started being hyped. So Caffe was really the dominant framework at the time and TensorFlow was on the horizon, but PyTorch was not quite on the horizon.
JK: Caffe can describe more complex models, especially when you go into training, and the engine we wanted at Tesla didn’t need to do that. So we built a simpler engine, but Ljubisa was already off to the races and he could show benchmarks and show good compute intensity. The Tenstorrent guys had a hacked-up version of their software that actually did something useful. It was paying attention to hardware/software boundaries even way early on in the design, and I thought that was key.
LB: We had a box that we were taking around and showing everybody in June 2016. It was running neural networks end-to-end on Caffe on an FPGA and returning results, and so it wasn’t anything that could sell but it showed that we could basically bring up this thing end-to-end relatively quickly and that we had a bunch of ideas. Ultimately it had all of our key focal points, even now, or essentially stuff we wanted from the get-go. It hasn’t been a huge revolution in high-level thinking since then, it’s been more just a massive amount of execution.
IC: The current outlay of Tenstorrent starts with the initial proof-of-concept Jawbridge design in 2019, with six Tensix cores and a small 1.5W design, leading into a bigger ‘Grayskull’ chip with 120 Tensix cores and PCIe 4. Grayskull is set to ship to customers this year. Most of the recent AI start-ups haven’t publicized their ‘proof-of-concept’ designs, so can you explain why it was important to have such a fast follow-on from this first mini-chip to the one that’s currently being sold?
LB: There were two reasons why we went about our product roadmap the way we did. One was purely risk management- it was a new team, no existing flows, no existing anything. We really had to flush the pipe and get something built so that all of the 50+ steps that you need to put in place to get it done can be done. We have it all working and we didn’t want to run a risk of sinking a pile of money in case there’s a mistake. The other motivation was that we believe pretty deeply that it’s important to have the same architecture basically span from edge to huge multi-chip, multi-computer deployments in the cloud. The main reason why we think you need an architecture that spans so widely is that as you go away from just running through a bunch of equations, to implementing the neural net, you get into more fancy things like runtime sparsity or dynamic computation or anything that tries to go away from the mindset.
Otherwise it becomes about just crunching a bunch of multiplies, the same multiplies every time, and you naturally run into compatibility dynamics. So imagine you end up with a situation where you train something on an NVIDIA GPU, and the hardware has a 2X sparsity feature in case you apply a certain set of constraints to your data. If you don’t have a compatible piece of hardware wherever you deploy this, either in a phone, or an edge device, you would lose that 2X. In terms of sparsity, that 2X factor is just going to grow anyway, in our view.
Essentially we felt it was a huge advantage to be able to clearly demonstrate that we can do a single watt chip. We can also do a 60-watt chip, and we can do a data center full of our chips connected through the Ethernet that’s also on our chips. So when you look at this spectrum, the single watt chip was the easiest to do, so you know, it was another point in favor of us doing it. It’s kind of a crawl-walk-run scenario, but shows the whole spectrum.
JK: You know, a lot of people spend a boatload of money on their first try. Grayskull (the chip shipping this year to customers) is our third-generation chip - we had that prototype in an FPGA as the first generation, and had the test chip as our second. The company has learned a shit-load on each step, both hardware and software. It’s reflected in the software stack, and our software team is pretty small.
I’ve seen a lot of AI companies who have got a chip back and their plan has been that to make it work they need to hire 300 software people. That means they don't really have a plan. You can’t really overcome that mismatch in the hardware/software boundary with huge teams. Well at some level you could, if you can throw 1000 people at it, and some people are doing that. That’s not really the right way to do it, and that’s not going to be something you could expose long run to programmers, because the complexities are so high, it gets really fragile, and then the programmers can’t see how the hardware works.
One of the key elements of Linux, as well as open-source software and running on x86, is that programmers could program the hardware right to the metal. They could see how it worked and it was obvious, it was robust, and it worked over time. For AI hardware that’s too fragile to be exposed to most programmers. Not all programmers, but you know, the ninjas.
IC: The nature of AI is naturally bi-furcated between training and inference, and the Greyskull chip is very much advertised as an inference design. With so many companies targeting the inference market, how much of a success has it been? What’s been the pickup rate between companies evaluating vs companies deploying?
JK: We’ve started production, but there’s a lead time on that. Our plan is really starting in Q3 and Q4 this year. We’ve talked to a whole bunch of people, and when we show them the benchmarks, they’re excited. It’s enough for them to ask for a box of hardware!
When we can run it and do it, we’ll see the pickup on the distribution. On the inferencing side, Ljubisa, what was your number? I think we could have made the chip about 20%-30% more efficient if it was inference only. That delta is small enough that if you’re going to go deploy a whole bunch of AI hardware, rather than have two different sets of hardware for inference and training, then the efficiency delta is small enough to maintain compatibility between the two. I think we did a good thing - Grayskull can do training, it’s going to benchmark really well on that, and with the next generation after that we have more networking between chips so we can scale up bigger and better. But they’re fundamentally the same architecture, making it easy to jump to the latest.
IC: Various AI chips out there are targeting ease-of-deployment with simplified code installation, single-core designs, peak TOPs, or consistent batch-1 performance. What makes the Grayskull processor the inference chip of choice for the customers?
LB: So purely on technical metrics, I think it stacks up very well versus other options that are available out there. The one thing that Grayskull can do, which I believe at least most of the alternatives that I look at cannot do, is conditional computation. This enables dynamic sparsity handling for AI.
So to make an example, if you’re doing training today, for the most part nobody can use sparsity at all. The way sparsity gets used is that usually you train a model with no assumption on sparsity. Eventually you post-process it after the fact, and you make a lot of weights for the model zero, and it becomes kind of a sparse model for inference.
So we’re able to use sparsity to our gain during training. For example, even during inference, where most folks focus on these weights, there is a trade-off in how many you’re going to prune out and make zeros against what kind of quality you’ll be left with. We’re able to make use of sparsity in intermediate results, in activations, in stuff that’s not known at compile time.
Finally we’re able to do relatively programmatic stuff in a neural network compute graph. We’re very good at having a bunch of heavy math-like matrix multiplies or convolutions or whatever, but if it’s followed by a node that does something that’s completely programmatic, we can do that on the chip. Our design is not all about math density, it’s a sword – it enables more general programming. Every one of our cores has a set of RISC engines in it which can basically run whatever you want on them.
So we believe pretty deeply that as neural nets continue evolving, both the dynamic sparsity and conditional computation, as well as programming the graph, are going to be getting more and more important. This is primarily because just putting in a bunch of expensive math has led to the largest models to be data-center size, but then if you don’t scale with any smarts, it is kind of difficult to sustain.
IC: By having this conditional computation in each of your cores, you’re sacrificing some die area. But you get more flexibility within the compute stack within the model application?
LB: You get the flexibility, definitely. Adding programmability isn’t free as usual, so there’s a bit of sacrifice there. But when you look at the raw numbers, let’s say the area of those RISC cores compared to the rest of the block, is below 5%. Power consumption is the same kind of story. Ultimately if you play your cards right, what you pay doesn’t have to be hugely noticeable in the die area of everything that goes into this design. We think we strike a good balance.
IC: What verticals have been interested so far: cloud service providers, government, commercial?
JK: I think there's another way to look at it. The three big areas of AI today are image processing, language processing and recommendation engines. Then there's also this movement from convolutional networks to transformer networks. Everybody's using the same building blocks. Then what we've noticed is a lot of people go and aim at the big hyperscalers, but the hyperscalers won’t deploy them until you can sell them a million parts. But they don't want to buy a million parts until they see a proof-point of 100,000. Those customers who want 100,000 want to see a 10,000-unit deployment.
We've talked to a whole bunch of people, from the top to the bottom of the stack, and they're all interested. The ones that are easiest to talk to that are going to move the fastest, like AI start-ups, or research labs inside of big companies that own their own software. They understand their models and just get on with it. Our initial target isn't to get some huge contract, it is to get 100 programmers using our hardware, programming it, and living with it every day. That's where I want to get to in the short run, and that's basically our initial plan.
But they are a fairly diverse set of people that we're talking to. There's the autonomous crowd, control systems, imaging, language. We're building this cool recommendation engine, which has an extra board and a computer with a huge amount of memory to make that model work. So it's fairly diverse, but we're looking for people who are agile, as opposed to any particular vertical.
IC: Jim has been quoted as saying that the Tenstorrent design was ‘The Most Promising Architecture Out There’. Can you shed some light on what this meant at the time Jim initially invested? Was that more about the initial Grayskull inference design, or was there vision into the Wormhole processor (next generation)?
JK: I would start with our flow. We have a compiler stack that starts with PyTorch generating a graph, and then the graph gets parallelized into smaller chunks. Those chunks have to coordinate between their computation, and then they execute kernels. That's the basic flow that everybody is doing. What we have is a really nice hardware abstraction layer between the hardware and the software that makes all that work, and that’s what I really like.
[In the world of AI], if you make a really big matrix multiplier, the problem is that the bigger it gets, the less power efficient it gets because you have to move data farther. So you don't want the engine to be too big, you want it to be small enough to be efficient, but you don’t want it to be too small, because then the chunk of data it's working on isn't that interesting. So we picked a pretty good size of what we call our Tensix processor. The processor is pretty programmable. It's really good at doing computation locally in memory, and then forwarding the data from Compute Engine to Compute Engine, in a way that's not software disastrous.
I've seen people say that they have a DMA engine, and you write all this code for it, but then they just end up spending their whole life debugging corner cases. [At Tenstorrent] we have a really nice abstraction in the hardware that says (i) do compute, (ii) when you need to send data to put the data in the pipe, (iii) when you push it in the pipe, the other end pulls it out of the pipe. It's very clean. That has resulted in a fairly small software team, and software you can actually understand.
So when I say that it is promising, it is because they have got a whole bunch of things right. The compute engines are the right size, it natively does matrix multiply and convolution (rather than writing for threads), and it natively knows how to communicate data around. It's very good at keeping all the data on-chip. So we're much more efficient on memory - we don't need HBM to go fast!
Then when we hook up multiple chips, the communication on a single chip compared to the communication from chip to chip is not any different at the software layer. So while the physical transport of data is different on-chip with a NOC versus off-chip with Ethernet, the hardware is built so that it looks like it is just sending data from one engine to another engine - the software doesn't care. The only thing that does is the compiler, which knows the latency and bandwidth is a little different between the two.
But again, the abstraction layers are built properly, which means you don't have to have three different software stacks. If you write on a GPU, you have to be a CUDA programmer in the thread, then you have to coordinate within the streaming multiprocessor, then coordinate on the chip, then you have NVLink which is a different thing, and then you have the network which is a different thing. There are many different software layers in that model, and if you have 1000 people, or if that's what you think is fun, that's cool.
But if you just want to write AI software, you don't want to know about all those different layers. We have a plan that actually will work.
We're also doing some other interesting things. We are adding general-purpose compute as part of the graph and future products, and we're looking at how to make it programmer-friendly. We’re also asking programmers want they want to do. I've done a lot of projects where you build the hardware, and then the software guys don’t like it because that's not what they wanted. We are trying to meet the software guys where they are at, because they just want to write code. They also want to understand the hardware so they can drive it, but they don't want to be tortured by it.
IC: One of the things when I speak to AI software companies is that when they go from a computation on-chip to a multi-chip approach, you have to break down the graph to be able to parallelize it across multiple chips. It sounds like in your design, or in the next-generation Wormhole design at least, you have 16 x 100 gigabit Ethernet connections per chip to connect to multiple chips. From what I understand here, the programmer's perspective doesn’t change if they’re working on one Wormhole or 100,000? The compilation is done, and the software stack is still just the same?
JK: Yes! We're actually building a 4U server box with 16 Grayskulls in it. For Greyskull we don’t have Ethernet but they're connected with PCI Express Gen 4. The compiler can break down a graph across those 16 parts. The software doesn't care if the transport is PCIe or Ethernet, but Ethernet is more scalable in a big system so we switched to Ethernet for our next generation more scalable part. But we'll be able to take a training model, have it automatically compile down the software will do the graph, not the programmer.
IC: When you scale to multiple chips, there's obviously going to be a latency penalty difference when you're moving data between Tensix cores on-chip compared to off-chip. How does that factor into your design, and then from a software level, should the programmer care?
JK: Here's the interesting thing about the computation. Matrix multiply is in general N3 computation over N2 elements - as the footprint of the computation gets bigger, the ratio of bandwidth goes down. We think we have the right ratio of bandwidth on Wormhole (next-gen 2022) so that the computation can be very effectively utilized on chips well as the 16 Ethernet ports that supports the communication between chips.
Then the problem comes down to the graph. As a solution designer, you have to think about how to decompose the problem and then how to coordinate it. That's all handled by our software stack - the programmer doesn't have to be involved in that. The programmer can think more about how they want their model to look, and the compiler takes care of how to get the best rate out on the chips.
IC: For both the current 2021 Grayskull chip and the future 2022 Wormhole chip, they're kind of in this sort of 65 to 75 Watt boundary. As you scale out to 1000s of chips, the communication across the whole array becomes a major part of the power discussion, right? By contrast, if you were to have made 300-watt chips would you be able to keep more on die, and have less power would be wasted on communication. How do you marry the two between having a lower power chip, but a wider network array?
JK: We’re more limited by die size. The Grayskull and Wormhole chips are actually fairly large. They’re on GlobalFoundries 12 nm. They are about 650 to 700 square millimeters?
LB: 620 mm2 (for Grayskull) and 670 mm2 (for Wormhole).
JK: So for Grayskull, we have a 75-watt card. That’s the chip plus the DRAM and everything else on the card (so it’s not the chip that’s 75 watts). But we could also run it at 150 watts, at a higher frequency, at a higher voltage.
Then for the next generation beyond Wormhole, when we do a process node shrink, we're raising the frequency quite a bit and the computational intensity goes up. We're also shifting to a higher bandwidth interconnect. It's a slightly higher power form factor, but it's still less than the current GPU kind of roadmap.
One of the things we do is since we keep most of the memory traffic on-die we don't need the high bandwidth memory, which has a high power cost. Then for the Ethernet stuff, you only use real power when you're communicating. We can do some dynamic power management, such that if the network ports are all fully loaded we can slow down the core a little bit to keep it balanced. So the numbers on the quote spreadsheet all look pretty good.
You know reality is fun, because we go build this stuff and we'll learn a lot. I've seen a lot as well, and I expect that we'll get our ass kicked on a couple things. We'll wish we'd done this or that different. But the design and simulations we have done look pretty good.
IC: Isn’t there always low-hanging fruit with every chip design though?
JK: Oh yeah, I’ve never done a project. Like I still remember, Pete Bannon and I were the architects of EV5, and when we were done it was the fastest microprocessor in the world. I was so embarrassed about what I did wrong [on that chip], I could barely talk about it. I knew every single problem. But they still put it in the Guinness Book of World Records. It's a funny phenomenon, when you're designing stuff, because you are intimate with the details - you talk about the cool stuff, but you know everything.
IC: The next generation Wormhole looks immense. You’ve called it a processor and a switch all in one, as the 16 x 100 GbE ports allow for seemingly unlimited scaling. The presentation at the Linley conference was quite impressive, showcasing 24 of these 75 W chips in a single rack unit, chip to chip enabled through 4 of those connections in a mesh, and then server to server with multiple terabits, and then rack to rack even more, while keeping the same native 100 GbE chip-to-chip connectivity regardless of location. What is the purpose of Wormhole?
LB: That’s a loaded question! It will depend a lot on the workload that you're running, and the sort of prototypical ‘mega-workload’ of today are these transformer models, like GPT-3. GPT-3 is probably the most famous member of that family right now, and the way people tend to organize those is by a bunch of replicated modules. In these modules there are encoders and decoders, and they're very similar. The model is a stack of these - a long stack of them. So for a model like that, you can really take the 2D mesh very, very far.
The questions that arise are whether the module or encoder fits on to your specific hardware architecture. So usually for most other architectures that we have been watching you want pretty high bandwidth communication within the encoder or within the decoder, and then the bandwidth needs on the boundary of these boxes are kind of more limited. So what has emerged are solutions where you will have a shelf which is like a 2U or a 4U, and you map one of these blocks onto them. Then there will be network communication between these computers, and they coincide with the edge boundaries there. So one of the messages we tried to give at Linley (the Linley Conference) was that the need to match the encoder or decoder module to a specific 2U/4U system is potentially detrimental. It's a boundary that forces model designers to adopt a certain set of sizes in a certain model architecture in order to fit the machine.
So one of the things we did was to remove that boundary. We created a uniform grid, it's really large, and you can basically place the entire pipeline onto that. There are no bandwidth cliffs, and there's nothing that you really need to be super worried about as you are designing models. One of the big messages that the guys at Linley tried to give was that we've attempted to remove the constraints from the model designers for this. There has been a kind of artificial limit to one box per one module of your model which has emerged. So as long as the models don't change the 2D mesh abstraction is likely to hold up pretty well. The models themselves are 2D-ish, and they're kind of left to right with data flow, so you don't see a lot of random connections skipping around from the beginning to the end of the model and so on. So as long as that holds the 2D mesh, it is a pretty good abstraction.
JK: You know, there's a funny thing - your brain is, I just read this recently, your cerebral cortex is 1.3 square feet. And it's essentially, it's a flat thin layer but only six neurons high, but it's built out of these columns of about 100,000 neurons, and it's very uniform. So it's a little 3D-ish. It's very big in two dimensions, but only six deep in the other dimension. That's been stable for, you know, hundreds of millions of years.
IC: But the brain has had hundreds of millions of iterations to get it to where it is today. There have also been hundreds of millions of iterations of getting it wrong.
JK: Yeah, that's for sure! That's why [at Tenstorrent] we're iterating every year, and we're going be at this for a while. It's pretty cool. The mathematics right now is being formulated as 2D matrices, and the 2D mesh is natural for that, and the way the graph compilers partition stuff is pretty natural for us. The N3/N2 works in our favor.
But getting rid of the artificial boundaries, such as only having eight chips and you might only design for eight chips, whereas we're building this really cool box with Wormhole, with a lot more chips in it. It has enough bandwidth that we can then make that as part of our mesh going forward. There are also trade-offs to think about, such as if or when one of the shelves breaks, and how do you repair it. Like, how do you want to build the mesh? People have lots of different opinions about that, and essentially at that level we're flexible, but yeah, there's something powerful about meshes. Then you have to do a lot of work on reliability and redundancy and rerouting, there are all kinds of interesting details on there.
IC: With the 2D mesh concept, it’s great that you bring up this six neuron high mini-3D model. Is there any desire or roadmap you think on AI compute to move to a more 3D style?
JK: It would be natural for us, because our Tensix cores are actually big enough that they could do the local 3D a little bit. So if somebody wanted to say they want a mesh to be a couple of layers deep, we could do that pretty naturally. For full three-dimensional, we'd have to really think about how the graph partition worked. But you can also plot a 3D thing onto a 2D thing.
IC: Where do you see Wormhole’s limits as the demands on AI grow? Localized SRAM, on-chip memory, compute-per-Tensix core, or bandwidth, or something else?
JK: That’s a pretty good question.
LB: As things stand, with today's crop of workloads it is pretty well balanced. It's somewhat difficult to predict what is the first parameter that we're going to end up tweaking as things change. Between Wormhole and Grayskull, we've actually changed the mix of compute and memory in the newer core, so we have more memory per core, and we have more compute per core than before, as well as a little fewer cores per chip. Ultimately that was kind of driven by just literally measurements. What we see as we compile workloads has evolved between the two chips. Your point is definitely true and that workloads evolve, and the right balance of compute to memory to I/O bandwidth also is going to evolve, but what exactly is going to change first? It's kind of hard to predict.
IC: In one of the diagrams in your recent Linley presentation Ljubisa, you have a rack of Wormholes, and then you have another rack of Wormholes, and the idea is that you span out multiple racks of Wormholes, all connected by 100 gigabit Ethernet. We're talking 100s of connections server to server and rack to rack. At what point does the cost of the cables become more than the cost of the chips, because those cables are expensive!
LB: We’re not there yet! Jim made a funny remark when we reviewed our internal Wormhole system a couple of weeks back. In the Wormhole systems we don’t have any host processors, so there isn’t any Intel chip in there, or any AMD chip in there, running any kind of Linux. Jim looked at the BOM (bill of materials) cost for the machine and he goes ‘well, I guess we cut out the processor costs, but replaced it with cables’. [laughs] They’re a non-trivial piece of the cost at this point, but they’re still very far from reaching to the level (in cost) that the machine learning processors are at.
JK: That's one reason why power density really matters. We're building this really dense 4U box partly to save cables. It definitely matters. On the flip side, networking has gotten better! I remember when we're struggling like crazy to get to 10 gigabit, and then 25 gigabits a second. We've raced through 50 gigabit, 100 gigabit, and 400 gigabit because of signal processing on the wires and just a whole bunch of technology innovation. Network bandwidth has gone up a lot, and it's amazing that this layer two transport layer which worked at 100 megabits is still a pretty good answer at 400 gigabits. It's a really interesting technology when you nail the abstraction layer. How far can go? I always joke that they must have changed the laws of physics, because I remember when 100 megabits was a pain, and now we have 400 gigabits.
IC: A few companies have been saying the future is along the integrated silicon photonics line, but the companies that are doing that have multi-billion dollar budgets. Is something like that viable for a start-up like Tenstorrent?
JK: Photonics has been the thing that ‘we're going to have to go to in five years’ for 20 years. It's another one of those interesting phenomena - it's harder to build, especially when the pace of innovation on copper has been spectacular. The first version of photonics will be when is it better in the rack to use photonics than copper, not whether it has to go to the chip. The answer still depends on how far you're going, and how much bandwidth you have. They're starting to do the top of the rack mega pipes with photonics. But the wires in a rack are all copper, and the economics of the solution are really clear. It's one of those static things. It's like people said ‘Moore's Law is dead in 10 years’. When I realized they've been saying that for 20 years, I decided to ignore it, or at some point investigate why people had that belief for such a long period of time. It's actually biblical apocryphal stuff. The world is always going to end in 10 years, because that's when the diminishing return curve runs out.
IC: So what is the future of Tenstorrent’s designs? You’re going from Grayskull in 2021 to Wormhole in 2021, to the gen after that - I think Jim alluded to more compute, to more networking and interconnect. What’s the direction here?
JK: We just announced with SiFive that we're licensing their cores, and on the next generation we’re putting an array of processors in there. We’re doing it for three reasons. It enables local network stack kind of stuff, such as Smart-NIC behavior and some other things like that. These cores can also run general-purpose Linux, and so you might want to just locally compute some kind of data transformation while you're using the Tensix cores. But the interesting thing from an AI perspective is that we can put bigger CPUs inside the compute graph on each chip. So as you're computing some result, you may want to run a C program on that data, and it's bigger than what the core can do locally, so we can give it to a bigger computer inside the same chip.
Again, it's about how to give programmers what they want. They want to build models, they want to write code, and they want it all to work together. They don't want to have this kind of archaic environment where there’s an accelerator and there's a host and this world is Linux and this world is something else. There are lots of boundaries in AI software today. We're trying to limit that kind of stuff. So we're doing it by adding general purpose compute and making sure that all the pieces work really well together.
IC: Those are SiFive’s X280 RISC-V cores, with 512-bit vector extensions?
JK: Yeah, there’s a nice big vector unit and it has the right data types that we wanted for the computation. So you can send the dense 16 bits load over there, and it can compute it, and has the nice big vector unit. They (SiFive) are doing a lot of work to make sure that if you write reasonable vectorized code it will compile and run pretty well. Chris Lattner is over at SiFive now, and he's one of the best compiler guys on the planet. So it gives us some confidence about where that software direction is going to go. I think RISC-V is really going to, well it's already making huge inroads all over the place, but with the next generation stuff with big vector units and really great software, RISC-V is going to be pretty cool.
IC: Do you take a die area hit implementing these extra RISC V cores – is it significant?
JK: In our first one (post Wormhole), no. We basically have an array of processors, and we are going to put a column in of CPUs per chip. It’s not big, but it has a lot of general-purpose compute, and now part of what we're doing is evolving our chip designs. We are also going to evolve our software stack, and we're willing to do experiments. Because this is a really fast-changing field, we think this is an architecturally interesting direction to go.
IC: Is there any chance that part of your designs can be used as sort of like, a mix and match? Can you have a mesh of previous generation hardware connected to next-generation hardware. Has that ever come up? Or do you think customers in this space will just bulk change from one to the next?
JK: The software we are building is designed to be as compatible as possible. So if you like Grayskull, and then you go to Wormhole, you can build a 16 Wormhole chip machine that works pretty similarly (on the same software), but it scales better. Then when we go to the next chip, all that software you created is going to work, but then if you want to add more functionality you can do that too. If you want to add more functionality, you’re going to want the new one.
But computation intensity is going up, network bandwidth is also going up. So every year you need a new competitive product, but in a semiconductor world you tend to sell parts for 2-4 years, because as you go into production the costs come down. You have to have a pricing strategy. We expect to keep selling the first-generation and second-generation, they're still good solid parts. But we'll keep working on new features for the next round.
IC: Tenstorrent is currently partnered with GlobalFoundries for the first couple of chips, Grayskull and Wormhole. Is it still going with GlobalFoundries moving forward, or are you developing relationships with other foundries?
LB: We are developing relationships with other foundries, primarily due to the desire to do some chips in the future in even lower geometries than what we’ve been using.
IC: One of the issues with VC-funded AI companies is the lack of a sufficient roadmap, or the need to acquire funding for that roadmap. How should investors look at Tenstorrent from that perspective?
LB: Our roadmap has been kind of fairly static and consistent over the years. We've been able to predict what we're going to do a few years out, and so far we've just been doing that. Luckily enough, we've also been able to acquire the funds that are needed to sustain it, so you could say that it's been roughly split up into epochs. We talked about the basement thing and the office in the bad part of town and those kind of coincided with amounts of funding. After that, we moved into an actual proper working office, there was another epoch with another set of funding. That's when we did our first chip, the test chip. So pretty much every chip has coincided with a round of funding, and in the first couple of years, they've coincided with a move as well.
We recently completed a fairly large round of funding, which we haven't quite, you know, publicized just yet, in fact, I think this is the first public conversation we're having about it (Tenstorrent has since announced a $200m funding round on May 20th). What I will say is that it completely enables our roadmap for a couple of years. It enables not only our next one, but a couple of our currently planned future chips. Essentially we're in a place where we should be able to deliver everything we're planning to deliver, and by that point, we're hoping that it's going to be self-sustaining.
JK: I'll give you a number - we did a test chip and two production chips (Jawbridge and Grayskull) for $40 million total, and we have a great software team. The genesis of it is really interesting. We have people from the FPGA world, from AI and HPC, and the combination of their talents and insight plus the hardware/software match – it all means that our software team is way smaller than anybody else’s, and our software stack is way more effective and efficient. So that has saved us a boatload of money - we don't have to announce that we're hiring 300 software people to make our hardware work because we made good architectural choices, both at the software and at the hardware level. That has made us effective.
The smaller geometry chips are more expensive to do, by about 2X. So it's going to raise our burn rate a bit. But we have a pretty good line-of-sight to being profitable on the money we've already raised, so we feel pretty good about that. That's partly because we've been efficient so far, and we're not carrying around big technical debt on software or hardware. I think that's a pretty big difference from some other AI start-ups.
IC: Did bringing Jim on as CTO cause additional interest in the company? I mean, not only from the likes of us in the press but from potential investors or customers?
LB: Absolutely! I mean it's exactly the same across the spectrum - Jim has a reputation of somebody who is both uniquely able to judge the quality of a technical solution, as well as understanding how you actually bring it out to customers to go sell it. He can go from a technical product or technical concept in an engineering proof-of-concept type story, to something that's actually shipping in large volume. I mean it's been a very binary thing, from zero to one, pretty much since Jim joined. We've been able to kind of get in the door much more easily at whole variety of places, not only the press, but investors and customers as well, for sure.
JK: I’ve worked at two start-ups, SiByte and then P.A. Semi. I’ve met literally hundreds of customers, selling processors. They were both network processors, and selling into embedded markets. But I’ve covered a very diverse space. I have a lot of experience of meeting with people and figuring out what they're actually doing, what they actually need, and then figuring out if we are doing that, and if we're not doing that, why aren't we doing it? Then think what should we do about it?
So it's been fun - I don't know how many people [I’ve talked to] since I've joined on, perhaps 30 to 40 different companies. They're really diverse, you know, there's the edge, the autonomous side, the datacenter people, there's image processing, there's big cloud computation. The space is really diverse. But they all come back to the same thing - compute intensity, the right amount of DRAM, the right amount of networking, and software that works. They really want to be able to do stuff.
We're talking to a couple of big companies who are frustrated with the current closed ecosystem, and they are programming companies, so they want to be able to program the hardware. We're also thinking that we want to program the hardware too, so what is the big problem? But there are lots of secrets in this business, so we're telling them about how our stuff works, and we're going to open up both the hardware and software specifications quite a bit. They are super excited about that, so we can get the door open, but the doors don't stay open unless you have something to say.
IC: So is Tenstorrent big enough to have the scope to work with a few very specific customers on their very specific workloads for the hardware? A big thing about AI companies is essentially assisting their customers with the hardware and helping them program it to get the best out of it. Where does Tenstorrent sit there?
LB: There are two sides to that question. One is how much individual ‘support’ and customization we can sustain. The other one is more of a technical architectural question. [It comes down to] how resistant to the need to be customized for every given use case. When you peel the onion, that feeds all the way into hardware, and the software doesn't exist in isolation. But it's like Jim mentioned earlier, we have a fairly small team, partly because we really didn't need a bigger team to do what we wanted, but also partly because big teams are actually hard. Managing a team of 300 people and effectively contributing to the same codebase is actually something that not too many people or places are capable of. So we tried very very hard, every step of the way, to make our software such that it can be maintained by a small amount of people, while still meeting the goals that the world was in front of us.
One of the side effects is that there's very little manual intervention when it comes to machine learning. If you bring in a neural network that we haven't seen before, we have tried to make it so that the likelihood that we really need to do a bunch of manual tweaking is low. Then if we do need to do it, it’s easier. Like that it's something we can get done in a couple of days, and not have a three-month burn on. I think we've materially succeeded there. So our software stack is pretty well structured, and pretty resilient to new workloads. It is also small - the volume of it is low. We're talking about a 50,000 lines of code kind of product, not a 5 million one. That makes it a bit easier.
On the other hand, there is still a bunch of work to do for each customer. People usually want to integrate into existing software stacks, into solutions that span more than machine learning. For example, you have video coming in on a camera, and whoever wants to buy an inference engine for that video they want to integrate it into their pipeline. So they want video coming in over IP, decoding it, feeding it into us, decorating it with boxes, or conclusions, re-encoding it, potentially connecting to payment platforms, all sorts of ancillary stuff that's completely unrelated to machine learning.
So for this sort of thing we are building up a team internally to support this sort of work. That's kind of part of the solution, and we will certainly be able to sustain this kind of work with a few large customers. But in the long term you really need to build up an ecosystem of collaborators for this kind of thing. I don't think even the heavyweight companies in this space actually pull all of this by themselves. Usually it's a bit of an ecosystem-building exercise, and we have that in our plans, but it's a step-by-step process. First you have to really crush the machine learning workloads, convince everybody that you've done that, and you have something great. Then you kind of throw a lot of effort into building an ecosystem, so all of that’s coming down the pipe.
JK: We want to scale the support end of it, not the core compiler side. If we do that part right, it should be a really good tight team. And I saw this at SiByte - there were three operating systems we had to support: the Cisco operating system, Linux and Wind River. Then we had to set the drivers on top of that, and once we nailed that picture, lots of different people could do all kinds of stuff. Because once the broad support package is there, they're then adding their own software on top of that platform which was pretty straightforward. We had 100 customers with a small support team, but until we figured out the mechanics of the pieces, it seemed complicated, because everybody seems to want something different. But once we got the abstraction layer right in the right operating system and the right set of drivers, then a lot of people could do different things with it.
IC: Speaking of personnel, at AMD Jim was the boss and Ljubisa was part of Jim's team. This time around, it’s Ljubisa that’s the CEO, and Jim’s the underling. How is that dynamic working? Is it better or worse? How does it come across day-to-day?
LB: That’s not an accurate portrayal of the way we have it set up! We’ve agreed to do this thing as partners for the most part. So Jim is certainly not an underling to me. In reality, if anything, you know, Jim’s somebody that’s senior to me in every way conceivable. So I think it’s cool that we’re doing this in partnership, but really if anybody’s kind of taking direction at points or ambiguities, it’s me.
JK: Ljubisa created Tenstorrent and his team, his original founders and then the core team are some really great people there. We talked a lot about what we should do, and you know, I think it would be absolutely great if we IPO and Ljubisa’s the CEO, and I'm supporting that 100%. We get along really good, we talk every day, and there are some things we divide and conquer. He's been really focused on the software stack, and I’m focused on the next generation part. There's a bunch of architectural features that we work on - we get together, we talk about it, and we say ‘you do this, I'll do that’. So far, that's been working really well. There's some business stuff that I've been focused on a lot more, because I'm really interested in how this is going to go together and go to market. But yeah, and with investors we mostly both talk to them, then that's pretty good, and we cover different areas. So you know, so far, so good.
IC: That’s actually quite good because it leads me onto asking Jim about what exactly what you’ve be doing at Tenstorrent so far. At the point you joined, Wormhole was already in the process of going to fabs, being taped out, or at least in the final stages of design. So in my mind you’re working on the next generation and the generation after that, but now you’re telling me you’re doing a lot of the business side as well?
LB: Jim’s a great sales guy. I always figured him to be a persistent dude, somebody that knows technology, but frankly I was surprised by his level of comfort in these meetings with customers.
JK: I was at two start-ups where we had a really good team, with a guy could get all the doors open. We had me that was talking about architecture, with a software guy, with a system board guy, we just had a team. We would go talk to people and go solve their problems, and when you do that, you learn a lot. The thing I like is ‘oh, that's what you're doing with our chip! Holy crap, we weren't thinking about that at all!’.
I've been told at various points in my career to focus on that high-level picture of managing, but I always like to get into the details, because that's where you learn everything, and then you integrate it together and then do something with it. So talking to customers is fun, especially if they're smart and they're trying to do something new, so I like to do that kind of stuff. I like to work in partnerships, you know, like Pete Bannon and I have been working together on and off for 30 years. We worked on the VAX 8800, EV5, we worked at P.A Semi and then Tesla, and Apple. Jesus, is that how many places [with Pete]! Dirk Meyer and I worked on at DEC and AMD together for years. You know, I play pretty well with others!
It’s only recently that I found myself being the VP. Raja Koduri and I worked together when he was at AMD, and I was at Apple. Then he came to Apple, and then I went to AMD, then he went back to AMD. He went to Intel, and then I went to Intel. So you know we worked on a whole bunch of projects together so I'm pretty used to, I would say, ‘intense’ intellectual collaboration. Then you find you’re sort of good at different things - like Dirk was way better at execution and details, and I would come up with crazy ideas. He would look at me like ‘I don't know why you want to do that, but I'll try it’. We had a lot of fun doing stuff. I wrote the HyperTransport spec, and the original spec was like 16 pages. I sent it to Dirk, and Dirk said ‘Jim, I know how you think – do you mind if I fill in a few details?’. Three days later I got a 60-page spec back that actually looked like something that a human being could read. He was 100% right, because he actually did know what people needed to see in a spec. So yeah, I like that kind of stuff, it's really fun.
IC: I think you have cultivated that image of mad technical scientist - let’s try a bunch of stuff and somebody else can fill me in with the details, but let’s fill in a bunch of stuff. I mean how often these days do you find yourself in the nuts and bolts of the design versus that more sort of holistic roadmap?
JK: All the time.
IC: All the time?
JK: Yeah, I don’t want to go into too many details, but when I was at Intel, people were surprised a Senior VP was grilling people on how they manage their batch queue, or what the PDK is, or what the qualification steps were, or how long the CAD flows took to run, or what the dense utilization was in the CPU, or how the register file was built. I know details about everything, and you know I care about them, I actually really care. I want them to look good.
A friend of mine said, if it doesn't look good, it isn’t good. So when you look at the layout of a processor, or how something is built, it's got to be great. You can't make it great if you don't know what you're doing. It’s bad if somebody gives you like a five-level abstraction PowerPoint slide that said, things are going good, and we're improving things by 3% per year. I've been in meetings where somebody said 5% better, and I said ‘better than what? Better than last year, or better than the best possible, or better than your first-grade project?’. They literally didn't know what - they'd been putting 5% better on the slide for so long, they forgot what it was. So yeah, you have to get the details.
If you're going to be in the technology business in any way, shape, or form, and you have to get to the details. I thought I was good at that, and then I met Elon Musk. Holy crap. For him the details started at atoms. Maybe lower, I don't know. But like, what I thought was first principle thinking wasn't close to his first principle thinking, and then I got my ass kicked seriously about doing that. But it was really great, I really like to do that. I hope that when I engage with engineering teams, they start to get that engineering is fun, and the details matter, and there's an abstraction stack - there's the high level, there's the medium, and there's a low level. Yes, you do need to know a lot about all of them, because then you can figure out how to fix things. You can't fix something simple like ‘computer too slow’ - what are you going to do if it's too slow? If you could go into 1000 details, there's all kinds of stuff to do if you know the details.
IC: I’m surprised that people would wonder why you’re asking detailed questions about, register file and cache use and such, because this is stuff that you’ve been doing for so long. It kind of seems weird to me, it’s almost as if the person you were speaking to didn’t know who you were?
JK: Yeah, but the positions get associated with technical level. My team at Intel was 10,000 people, right, so you spend a lot of time on organization charts and budgets, and all kinds of admin, and then it's easy to find yourself just doing that and saying you’ll hand off leadership for a project to this person or this person and that. But the problem is there's so many things that are cross-functional – I want to know what the fab is doing, how does the PDK work, how does the library work, how does the IP team work, how does the SoC integration work, how does the performance model work, how does the software work. Then you find out that if you can't deep dive into all those pieces, bad things happen.
My father worked in GE when Jack Welch ran GE, and he said Jack could go to any part of GE and within a day and get to the bottom of it. He got credited with a whole bunch of business innovation, but I heard from many people that Jack could get to the bottom of anything. I read his book Straight From the Gut years ago, and I thought, well, I'd like to be that kind of person you know - if I'm going to manage people, I'd like to be the kind of person to get the bottom of anything.
IC: It’s well known that you’ve been bouncing from company to company every few years….
JK: Nooo, that’s a myth!
IC: That is the general perception, so it have to ask, because Ljubisa alluded to your age earlier, as being more senior - is Tenstorrent your final home, as it were? When do you see yourself retiring?
JK: I'm going to be here forever, but I probably will have a few other things going on. And, you know, I was talking to some friends about a quantum qubit company, which I think is hilarious. Then I have a couple of other friends where we've been brainstorming on how to do a new kind of semiconductor start-up, that's like a million times cheaper than the current stuff. There's other stuff I'm interested in, but at Tenstorrent, our mission is to go build AI for everyone - programmable chips that people can actually use. That's a many-year effort.
IC: So will you ever retire?
JK: I read a book somewhere that from retirement to death is 10 years, and I want to live to be 100, so I’ll retire at 90, I guess?
IC: You hear that Ljubisa, you've got Jim until he’s 90.
LB: That sounds great! It’s not a surprise, we haven’t discussed it, but if you ask me I would have said something similar.
JK: I like to do stuff - I snowboard with my kids, and I go kite surfing in Hawaii. I like to run and goof around. I've got stuff to do, but I like to work and I like technology, and it's really interesting. It's amazing, you know that periodically people think this day is the end of this, or the end of that, and holy cats you know the AI revolution is bigger than the internet. It's going to change how we think about everything, how we think about programming, how we think about computing, how we think about graphics, images, all kinds of stuff. So yeah, it's a really interesting time to be part of technology.
IC: Is there anything about Tenstorrent that you want people to know that hasn’t really been discussed anywhere?
JK: Well, we're hiring. So if people want to! I really love engineers who would love to do stuff that are really hands-on. I hired a bunch of senior CPU guys, and the first thing they did is they started bringing up the DB infrastructure and building a model, and getting to the bottom of it. So you know if you want to manage a bunch of people and sit around making PowerPoints, please don't apply to us. But if you're a technologist and you want to be hands-on and do real work, that's great. Or if you're young and smart, you want to work hard and learn something from really good people, do it. How many people were on the team that built Grayskull? We built a 700-millimeter world-class AI chip with 15 people?
LB: That's right.
JK: It's unbelievable. So if you want to learn how to build stuff, this would be a good place. So send us your resume. They finally prodded me to join LinkedIn. I don't really know how that works, but they tell me that's going to help me connect with people and hire.
IC: It really doesn’t!
JK: I don't know! The thing I really like is it keeps saying ‘your network says you know this person’ - I was like I worked with him a couple years ago, and he was great. So I sent him a message, ‘hey, you want to come work with us?’ It's been kind of fun, it's like a little memory lane walk for me because of how the algorithm works. But yeah, I'm not a social media person at all. I just like to talk about things, and I think I've sent out like three tweets in my whole life. It’s pretty fun.
IC: You are in Toronto, Austin, and the Bay Area right now?
JK: That’s right, yeah, we did. We took a brand new office in Santa Clara. 10,000 square feet, it’s beautiful. We have an offer on a new office in Austin, which is going to be great. I like having a really nice place to work. When I go to work, I want it to look good. I still remember when I went to Apple, it was hilarious. They changed the font on one of the Mac manuals, so they repainted the fonts on all the signs around the campus to match. That attention to detail just cracks me up, but it was a really nice place to work and it was cool. So yeah, we have three offices, which are all going to be nice places to work.
Many thanks to Ljubisa, Jim, and Christine for their time.
Also thanks to Gavin Bonshor for the initial transcription.
130 Comments
View All Comments
ralritt - Thursday, May 27, 2021 - link
Great Kim Jeller interview!mode_13h - Friday, May 28, 2021 - link
Absolutely! This is like a treasure trove of all kinds of details we rarely ever hear!I really have a lot more respect for Jim, after reading this. I wish nothing but the best for them.
GeoffreyA - Saturday, May 29, 2021 - link
It was great to get a glimpse of Jim's personality, and one sees he's such a likable, down-to-earth man. A lot of humility too.As for the "treasure trove," goodness gracious me, it's overflowing!
outsideloop - Thursday, May 27, 2021 - link
I would love to see an interview with Mike Clark in the future, Ian.Ian Cutress - Thursday, May 27, 2021 - link
You'd be surprised how many companies are uncomfortable with full interviews with engineers. Executives are fine, but engineers can be more 'loose' with answers and say something they shouldn't. Also if an engineer does a very good interview, they might be highlighted to competitors, and the company doesn't want the engineer poached.mode_13h - Thursday, May 27, 2021 - link
Just curious: what's the deal with the trio of portraits, about a page in? I found it confusing, since the top of the article has actual photos of them, and any readers of this site or your twitter feed will have seen your pic. Also, the difference in styles is a little jarring.No big deal, but it had me wondering.
Ian Cutress - Thursday, May 27, 2021 - link
I usually put the photos in for people who read the article in systems where the top carousel image doesn't appear. The number of photos of Jim and Ljubisa in the wild is quite small - Tenstorrent provided the drawn portraits as even they don't have recent headshots. I had to do something with my photo so it wasn't just the regular picture, that would look more out of place imo.Fataliity - Thursday, May 27, 2021 - link
What is your timeline for releasing the second half of this article? Soon? Or like a few months?Spunjji - Friday, May 28, 2021 - link
I enjoyed seeing you rendered as what appears to be an incorporeal being constructed from discrete data vectors 😅GeoffreyA - Friday, May 28, 2021 - link
Best picture. Sort of reminded me of the Dahaka from Warrior Within.